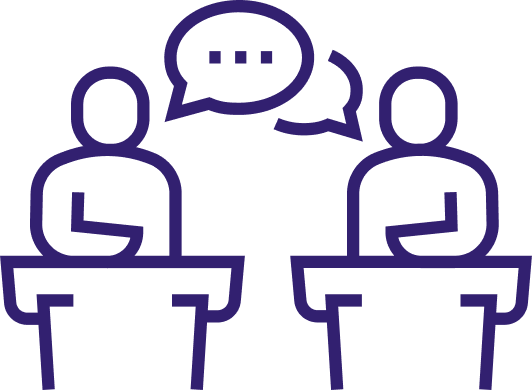
February 10, 2024 | 6 min read
Event Summary
U.S. Senate Finance Committee – AI and Health Care: Promise and Pitfalls
Table of Contents
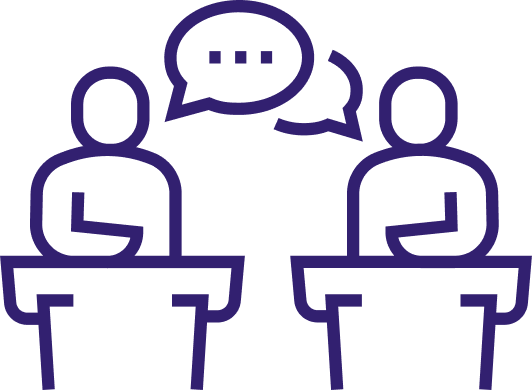
Executive Summary
Thursday, February 8, 2024 | 10:00 am – 12:00 pm ET
On February 8, the U.S. Senate Committee on Finance held a full committee hearing to detail the role Congress and the Committee should play to ensure positive outcomes and set rules for AI-supported innovations in the American healthcare system.
Key Takeaways 💡
- Challenges in the implementation of AI in healthcare include a lack of transparency and reimbursement structures, concerns over patient privacy and discriminatory bias, and healthcare organizations not having robust review processes.
- There needs to be better reimbursement structures for AI models and devices from CMS to help guarantee financial support. Inconsistent and unpredictable reimbursement stifles adoption by providers, especially in rural and underserved areas.
- Guardrails are only applicable to the large healthcare organizations that have adopted AI. Congress should ensure all organizations can adopt AI, potentially using government funding of EHR adoption as a guide. Governance must focus on the algorithm and how the algorithm is integrated into the clinical workflow.
Members of the Committee
- Ron Wyden (D-OR), Chairman of the US Senate Committee on Finance
- Mike Crapo (R-ID), Ranking Member of the US Senate Committee on Finance
- All members
Witnesses
- Peter Shen, Head of Digital & Automation for North America at Siemens Healthineers
- Mark Sendak, Co-Lead at Health AI Partnership
- Michelle M. Mello, Professor of Health Policy and of Law at Stanford University
- Ziad Obermeyer, Associate Professor + Blue Cross of California Distinguished Professor at UC Berkeley
- Katherine Baicker, Provost of the University of Chicago
Discussion Summary
How AI is Being Used in Healthcare
- Medicine requires processing large amounts of information, much of which could be imperfect—and mistakes have significant consequences on patient’s well-being and livelihood. AI can reduce decision-making stress by analyzing and making vast amounts of information more accessible to physicians and medical specialists. Through its predictive value, algorithms can help decide who needs what treatment, helping with distributing resources and time to patients.
- Siemens Healthineers has been applying AI to medical technology for more than 20 years through applications in imaging, diagnostics, and therapies. Their FDA-cleared devices that use AI or machine learning to produce clinical outputs for physicians to use in diagnosis—are intended to augment the jobs of specialists, not replace them, by providing them with better information to make a more accurate and informed diagnosis and/or treatment.
- Duke Institute for Health Innovation was the first to implement a deep learning model in routine clinical care and create Model Facts labels – similar to nutritional labels – for AI tools. They have also utilized AI in sepsis care and chronic care management.
What Needs to Be Fixed?
- There must be transparency in how tools are being developed and used. This is vital to foster trust, ensure accountability for how they are used, and protect the privacy of patients.
- There have been some rules proposed by the FDA and the Office of National Coordinator for Health IT (ONC) in the past few years but the regulation and governance must develop further and more robustly—something that the U.S. Senate Committee of Finance wishes to push from a bipartisan angle.
- There needs to be better reimbursement structures for AI models and devices from CMS to help guarantee financial support. Inconsistent and unpredictable reimbursement stifles adoption by providers, especially in rural and underserved areas.
- AI can have underlying bias and discriminatory analyses.
- For instance, Dr. Obermeyer and his team found a subtle-seeming choice in the AI’s design that was causing harm: a gap between what the algorithms were supposed to predict (health care needs) and what they actually predicted (health care costs). The AI was designed to identify patients with high future health needs. But AI is extremely literal—it predicts a specific variable, in a specific dataset—and there is no variable available called ‘future health needs.’ So instead, the AI developers chose to predict a proxy variable that is present in health datasets: future healthcare costs. He found that the tool, on average, required black patients to present with worse symptoms than white patients to qualify for the same level of care.
- Similar algorithms are still being used today and upwards of 150 million patients every year are being affected by them.
- AI tools must be supervised and monitored to ensure they are furthering equity—not furthering bias. Hospitals understand that they need to vet AI tools before use, but most healthcare organizations do not have robust review processes yet.
- For instance, Dr. Obermeyer and his team found a subtle-seeming choice in the AI’s design that was causing harm: a gap between what the algorithms were supposed to predict (health care needs) and what they actually predicted (health care costs). The AI was designed to identify patients with high future health needs. But AI is extremely literal—it predicts a specific variable, in a specific dataset—and there is no variable available called ‘future health needs.’ So instead, the AI developers chose to predict a proxy variable that is present in health datasets: future healthcare costs. He found that the tool, on average, required black patients to present with worse symptoms than white patients to qualify for the same level of care.
- There are also concerns about health plans using algorithms to automate the payment or denial of insurance claims. CMS addressed this in its most recent Medicare Advantage rule, requiring automated denials be in compliance with all applicable coverage determination rules. Sometimes that requires a review by a medical professional.
How to Govern AI
- Health AI Partnership, a collaborative initiative by Duke’s Institute for Health Innovation, helps to surface and disseminate AI best practices for industry players through webinars, guides, and workshops.
- These best practices can be used as guardrails by hospitals and be required for Medicare participation. For instance, the Medicare Certification Process could be used to require that hospitals have a process for vetting AI tools before deployment and to continue monitoring them afterwards.
- The Algorithmic Accountability Act may help root out algorithm bias by requiring healthcare systems to regularly assess whether AI tools they develop/select are being used as intended and are not perpetuating harmful bias.
- The federal government should also work to foster this idea of a consensus-building process that brings experts together to create national consensus standards and processes for evaluating proposed uses of AI tools.
- Guardrails, though, only serve the few organizations already adopting AI. For those not on the road to adopting AI, the government should focus on infrastructure investments so all people in the US can benefit from AI in healthcare. This can be accomplished through technical assistance, technology infrastructure, and training programs—similar to how Congress has done this before during the implementation of EHRs 15 years ago.
- These best practices can be used as guardrails by hospitals and be required for Medicare participation. For instance, the Medicare Certification Process could be used to require that hospitals have a process for vetting AI tools before deployment and to continue monitoring them afterwards.
- The federal government needs to establish standards for organizational readiness and responsibility to use healthcare AI tools since the success of AI tools depends on the adopting organization’s ability to support them through vetting and monitoring. Specificity is important in achieving transparency (what is an algorithm predicting?), and accountability should be maintained by measuring the performance of the algorithm in diverse datasets.
- Measuring of algorithms must be done through diverse and extensive datasets to mitigate bias and ensure the algorithm works throughout the population.
- AI assurance labs and other similar initiatives can help develop consensus standards and perform certain evaluations of AI models. While some aspects of AI need to be done locally, federal regulations can still help organizations invest in making it happen while keeping up with these standards.
- Governance must focus on the algorithm and how the algorithm is integrated into the clinical workflow.
- Most discourse and regulation often focus on algorithms on their own, but much of how AI works depends on how it is used. Research shows that humans are prone to automation bias (i.e., over-relying on computerized decision support tools), and as such, there should be regulations and guides on how to incorporate algorithms and AI models well into the workspace.
- Government programs should be willing to pay for AI that generates value, pricing services based on the health services they provide. Better reimbursement systems and not paying for or accepting flawed products – using purchasing power to shape the market.
- Regulation overall needs to be adaptable—or else it can be irrelevant or chill innovation.
Last Updated on February 11, 2024
Contact Us
Have any questions? We'd love to hear from you.