4 days ago | 9 min read
Summary
House AI Task Force Report: Healthcare Applications Chapter
Table of Contents
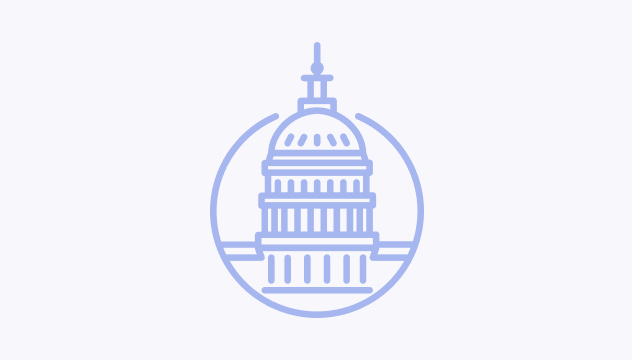
Overview
In February 2024, leaders from the U.S. House of Representatives convened a bipartisan task force to present recommendations for a future AI regulatory framework. The following is an executive summary of the healthcare chapter of the report, relevant findings, recommendations, and who contributed to the report.
EXECUTIVE SUMMARY
Led by Chairman Jay Obernolte (R-CA) and Co-Chairman Ted Lieu (D-CA), the Task Force published its final report on December 17, 2024. Over the course of ten months, the co-chairmen – along with several Democratic and Republican representatives and staff members – gathered information on AI issues. The report authors reviewed public, academic, and industry resources, held multiple hearings and roundtables, and engaged with over 100 AI experts to inform the content of the report.
The 253-page report includes 66 key findings and 85 recommendations, which a 20-page chapter on healthcare. Overall, it argues for a risk-based and flexible framework for AI regulation that addresses sector-specific concerns. Additionally, the authors claim that it would be “unreasonable” to expect Congress to pass meaningful legislation on AI this year, and that it is more likely that an incremental approach to AI regulation will be pursued – particularly to keep up with the fact that AI is constantly evolving.
The AI in Healthcare chapter includes two key findings:
- AI will help make the system more efficient, improve care and outcomes
- The lack of uniform standards is a challenge that must be addressed
The AI in Healthcare chapter includes five recommendations:
- Improve access to high-quality data
- Support AI-related biomedical research
- Create an evaluation process to vet and monitor health AI tools
- Develop liability standards
- Determine a reimbursement mechanism to support health AI innovation
In general, the chapter reviews the current potential for the use of AI in healthcare such as expediting drug discovery, reducing diagnostic errors, and alleviating administrative burden while also highlighting the concerns associated with the rapid adoption of these new technologies. Some of these concerns include a lack of transparency, potential bias, privacy risks, and the misuse of AI in payers’ coverage decisions.
Generally, the authors recommend that Congress increasingly monitor the adoption of AI in healthcare along with these risks. They also suggest that Congress may want to consider updating things like oversight of payer practices, the FDA’s ability to monitor these new technologies, standards that help evaluate AI’s safety and effectiveness, and CMS reimbursement frameworks.
Below is a summary of the report:
- Key Findings
- Recommendations
- Report Findings
- Relevant Contributors
Key Findings
- AI’s use in healthcare can potentially reduce administrative burdens and speed up drug development and clinical diagnosis. When used appropriately, these uses of AI could lead to increased efficiency, better patient care, and improved health outcomes.
- The lack of ubiquitous, uniform standards for medical data and algorithms impedes system interoperability and data sharing. If AI tools cannot easily connect with all relevant medical systems, their adoption and use could be impeded.
Recommendations
- Recommendation: Encourage the practices needed to ensure AI in healthcare is safe, transparent, and effective. Policymakers should promote collaboration between stakeholders to develop and adopt AI in healthcare where appropriate – this can be done through multidisciplinary workshops and conferences. Policymakers should also find ways to improve access to high-quality data that still protects patient information:
- “Examples include voluntary standards for collecting and sharing data, creating data commons, and using incentives to encourage data sharing of high-quality data held by public or private actors.”
- Also says Congress should monitor use of predictive technologies to approve or deny care and coverage.
- Recommendation: Maintain robust support for healthcare research related to AI, specifically research supported by the NIH. More on research/AI-related skills development in the Research, Development, and Standards chapter.
- Recommendation: Create incentives and guidance to encourage risk management of AI technologies in healthcare across various deployment conditions to support AI adoption and improve privacy, enhance security, and prevent disparate health outcomes. Standardized testing and voluntary guidelines that support the evaluation of AI technologies and help covered entities comply with HIPAA.
- Work with NIST on their AI risk management and evaluation frameworks
- Consider need for better guidance/regulation for industry post-market surveillance and self-validation
- Explore whether FDA’s post market evaluation process is sufficient, or current laws need to be enhanced to improve its ability to ensure technologies are being appropriately monitored for safety, efficacy, and reliability
- Recommendation: Support the development of standards for liability related to AI issues. Right now, there is no guidance on constructing legal and ehthical frameworks for liability regarding AI usage. Congress should examine liability laws to ensure patients are protected.
- Recommendation: Support appropriate payment mechanisms without stifling innovation. CMS’ current reimbursement mechanism is not adequate for most AI tools. There will be no “one size fits all policy” but there should be better mechanisms to adjust for different types of technologies. Congress should continue to evaluate new technologies and ensure Medicare benefits support them when appropriate.
Report Findings
The substantive aspect of the report described 1) AI adoption in the healthcare system, 2) health insurance decisions, and 3) challenges and risks related to AI in healthcare. Below is a summary of those three sections:
1. AI Adoption in the Healthcare System
- Drug Development – Acknowledges that AI is currently being used in drug development, and has the potential to decrease the time and cost of discovery, design, and testing of drug candidates.
- Fundamental Biomedical Research – AI, machine learning, and informatics are used to drive discoveries in fundamental biomedical research (e.g., understanding how proteins fold) which then in turn can be used by other AI tools for more innovation. This is especially pertinent with increased access to data through things like EHRs and wearables.
- Diagnostics – AI can help with diagnostics – both in relation to imaging and other clinical tests. Diagnostic errors are one of the largest drivers of harm and cost in the system. The potential for AI to help alleviate some of those errors exists, however AI must not replace clinicians in diagnosis – only supplement their decision-making.
- Clinical Decision-Making – AI tools may help augment patient care through treatment recommendations, population health management, or predicting patient health trajectories.
- Population Health Management – Outside of clinical decision support, these applications of AI can help stratify populations to identify health risks.
- Administrative Clinical Uses – Providers are increasingly using AI to help reduce administrative burden. This includes note-taking, EHR data population and documentation preparation for claims submission.
- If not implemented correctly in a way that accounts for potentially poor data that currently exists in the EHR, AI tools could degrade both the EHR and other medical documentation.
- Development of Medical Devices and Software – AI is changing the field of medical products, especially in the research and development of new therapeutics. The FDA Center for Devices and Radiological Health (CDRH) is increasingly reviewing AI-ML-enabled medical devices.
2. Health Insurance Decisions
The Task Force addressed how payment for using AI in healthcare is an unanswered question, particularly how reimbursement occurs for its use. Background research highlighted how CMS currently pays for some applications (like IDx-DR, a diabetic retinopathy diagnostic) through traditional coverage codes, however the current framework does not adequately address new technologies. The report recommends that Congress continue to monitor the situation and evaluate Medicare’s policies.
The report highlights how AI may be used to streamline fraud identification – as is being currently piloted by CMS.
The report also focuses on criticisms of health insurers for lacking transparency when AI is used in coverage decisions. There are some concerns that despite potential for streamlining administrative tasks, there is a larger concern over AI leading to unnecessary denials / barriers to treatments. Examples cited include:
- Questions raised about the use of AI systems to predict estimated lengths of stay, and rejecting patient requests for care that exceed that length.
- Citing that when denied claims of elderly patients claims for extended stays were appealed, 90% were reversed.
3. Policy challenges confronting AI in healthcare
- Data Availability, Utility, and Quality – AI systems must be trained on large data sets, which is not always high quality or able to be combined with other data. This is especially true in healthcare, where data is still siloed and lacks consistent standards. Federal research agencies also possess legacy datasets without guidance on how to organize, manage, or share their data with researchers – or when to sunset outdated datasets.
- Cultural concerns over health information sensitivity also will lead to a hesitancy to sharing data. De-identification is only so helpful, because sometimes the information that is removed in that process is necessary to ensure a model is being trained on representative populations appropriately.
- Transparency – Clinicians need transparency when AI is used in decision-making to be confident in the tools, and to build trust with patients. Lack of interpretability and explainability – that helps users understand how AI reaches its conclusions – will only exacerbate concerns over the use of AI in healthcare.
- Clinicians need training to understand when an AI tool may make a mistake, and to help prevent an under- or over-reliance on AI tools
- Bias – Bias can stem from algorithmic biases that occur when training data exhibits skew, bias, or a misrepresentation of specific populations. It may also stem from intentional or unintentional human bias that influences the design of the AI system.
- Privacy and Cybersecurity – AI tools require a lot of data to operate, meaning that patient data privacy is at higher risk. There are concerns about who has access to patient data and how it is being used, especially in post-Change Healthcare cyberattack landscape that revealed how significantly a breach can impact care delivery.
- HIPAA may or may not be relevant depending on who is controlling the health data.
- Interoperability – Despite investments, there is still a lack of interoperability – especially between EHRs, who may avoid facilitating data sharing. This may be an issue if an AI tool cannot access all the data sources it needs to advance its development.
- Liability – There is no legal or ethical guidance on accountability for when AI leads to incorrect diagnoses or harmful recommendations.
Relevant Contributors
According to the authors of the report, the task force met with more than one hundred experts and stakeholders to inform this research. The experts the authors met with to discuss healthcare applications include:
- Taha Kass-Hout, Chief Technology Officer, GE HealthCare
- Bradley Malin, Accenture Professor of Biomedical Informatics, Biostatistics, and Computer Science, Vanderbilt University
- Shannon Curtis, Assistant Director, Division of Federal Affairs, American Medical Association (AMA)
- Sara Murray, M.D., Chief Health AI Officer, University of California, San Francisco
- GAO’s Science, Technology Assessment, and Analytics Team
The authors also held roundtables with the following AI CEOs and experts:
- Sam Altman, Chief Executive Officer, OpenAI
- Jack Clark, Co-Founder and Head of Policy, Anthropic
- Alexandr Wang, Founder and Chief Executive Officer, ScaleAI
- Tom Siebel, Chairman and Chief Executive Officer, C3 AI
- Aidan Gomez, Co-Founder and Chief Executive Officer, Cohere
- Marc Andreessen, Co-Founder and General Partner, Andreessen Horowitz
- Max Tegmark, Professor of Physics, Massachusetts Institute of Technology, and President, Future of Life Institute
More Info
Please contact Maverick Health Policy if you or your team would like to learn more: paige.kobza@maverickhealthpolicy.com
Last Updated on December 17, 2024
Contact Us
Have any questions? We'd love to hear from you.